Our laboratory develops and applies computational approaches to study molecular variations and their phenotypic consequence. How does our genetic background shape phenotypic traits or cause disease? How are genetic and environmental factors integrated at different molecular layers, and how variable are molecular states between individual cells?
We use statistical inference and machine learning as our main tools to address these scientific questions. Growing sample sizes and technological advances demand novel analytical strategies and tools that scale to datasets with millions of observations and account for spatial and temporal dependencies. We develop foundational methods in statistics and machine learning, including efficient parameter inference in models to probe genetic associations and methods for dimensionality reduction.
Orcid: https://orcid.org/0000-0002-8818-7193
Google Scholar: https://scholar.google.com/citations?user=ClSXZ4IAAAAJ&hl=en

Computational Genetics
We develop statistical methods to dissect often overlooked dimensions of the genotype-phenotype relationship, including genetic effects of rare variants and indirect genetic effects.
Spatial and Multi-Omics
We develop foundational methods to integrate high-dimensional molecular profiles assayed in bulk or at the single-cell level. This includes innovations to allow for integrating multi-omics readouts across time and space, as well as methods for spatial omics technologies.


Causal Inference Models
We develop methods to model genetic perturbation effects and infer causality. We leverage opportunities provided by engineered perturbations using CRISPR screens combined with single-cell readouts.
Software
GitHub: https://github.com/PMBio/
GitHub: https://github.com/single-cell-genetics
Selected Publications
Computational Genetics
- Clarke, B., Holtkamp, E., Öztürk, H., Mück, M., Wahlberg, M., Meyer, K., Munzlinger, F., Brechtmann, F., Hölzlwimmer, F. R., Lindner, J., Chen, Z., Gagneur, J., & Stegle, O. Integration of variant annotations using deep set networks boosts rare variant association testing (2024). Nature Genetics [software] [publication]
- McCarthy, D.J. , Rostom, R. , Huang, Y., Kunz, D.J, Danecek, P., Bonder, M.J., Hagai,T., HipSci Consortium, Wang, W., Gaffney,D.J., Simons, B.D., Stegle, O. Teichmann, S.A. Cardelino: Integrating whole exomes and single-cell transcriptomes to reveal phenotypic impact of somatic variants (2020). Nature Methods [software] [publication]
- Moore, R., Casale, F.P. , Bonder, M.J., Horta, D., Franke, L., Barrosso, I., Stegle, O. A linear mixed-model approach to study multivariate gene–environment interactions (2019). Nature Genetics [software] [publication]
- Casale, F.P., Rakitsch, B., Lippert, C., Stegle, O. Efficient set tests for the genetic analysis of correlated traits (2015). Nature Methods [software] [publication]
- Jerber, J., Seaton, D.D., Cuomo, A.S., Kumasaka, N., …(9 additional authors) …, Marioni, J.C., Merkle, F.T., Gaffney, DJ., Stegle, O. Population-scale single-cell RNA-seq profiling across dopaminergic neuron differentiation (2021). Nature Genetics [software] [publication]
- Bonder, M.J., Craig, S., Gloudemans, M.J., Frésard, L., Jakubosky, D., D’Antonio, Xin Li, X., … (12 additional authors) …, Montgomery, S.B., Stegle, O. Systematic assessment of regulatory effects of human disease variants in pluripotent cells (2021). Nature Genetics [software] [publication]
- Cuomo, ASE., Seaton, DD., McCarthy, DJ., Martinez, I., Bonder, MJ., Garcia-Bernardo, J., Amatya, S., Madrigal, P., Isaacson, A., Buettner, F., Knights, A., Natarajan, KN., HiSci Consortium, Vallier, L. , Marioni, JC. , Chhatriwala, M. , Stegle, O. Single-cell RNA-sequencing of differentiating iPS cells reveals dynamic genetic effects on gene expression (2020). Nature Communications [software] [publication]
- Kilpinen, H., Goncalves, A….(35 additional authors)…,Watt, F., Durbin, R., Stegle, O., Gaffney, D.J. Common genetic variation drives molecular heterogeneity in human iPSCs (2017). Nature [software] [publication]
Spatial and Multi-Omics
- Marconato, L. , Palla, G. , Yamauchi, K. A. , Virshup, I., Heidari, E., Treis, T., Toth, M., Shrestha, R. B., Vöhringer, H., Huber, W., Gerstung, M., Moore, J. , Theis, F. J. , & Stegle, O. SpatialData: an open and universal data framework for spatial omics (2024). Nature Methods [software] [publication]
- Velten, B., Braunger, J.M., Argelaguet, R., Arnol, D., Wirbel, J., Bredikhin, D., Zeller, G., Stegle, O. Identifying temporal and spatial patterns of variation from multimodal data using MEFISTO (2022). Nature Methods [software] [publication]
- Kleshchevnikov, V., Shmatko, A., Dann, E., Aivazidis, A., King, H. W., Li, T., …, Stegle, O. , Bayraktar, O. A.. Cell2location maps fine-grained cell types in spatial transcriptomics (2022). Nature biotechnology [software] [publication]
- Argelaguet, R., Velten, B., …(4 additional authors)…, Buettner, F., Huber, W., Stegle, O. Multi-Omics factor analysis – a framework for unsupervised integration of multi-omic data sets (2018). Molecular Systems Biology [software] [publication]
- Svensson, V., Teichmann, S.A., Stegle, O. SpatialDE: identification of spatially variable genes (2018). Nature Methods [software] [publication]
- Angermueller, C., Clark, S.J., Lee, H.,J., Macaulay, I.C.,..(5 additional authors)…, Voet, T., Kelsey, G., Stegle, O., Reik, W. Parallel single-cell sequencing links transcriptional and epigenetic heterogeneity (2016). Nature Methods, 13, 229–232. [software] [publication]
- Buettner, F., Natarajan, K.N., Casale, F.P., … (4 additional authors),…, Marioni, J.C., Stegle, O. Computational analysis of cell-to-cell heterogeneity in single-cell RNA-sequencing data reveals hidden subpopulations of cells (2015). Nature Biotechnology [software] [publication]
Causal Inference Models
- Rohbeck, M., Clarke, B., Mikulik, K., Pettet, A., Stegle, O., & Ueltzhöffer, K. (2024). Bicycle: Intervention-Based Causal Discovery with Cycles. Proceedings of Machine Learning Research. 3rd Conference on Causal Learning and Reasoning 2024 [software] [publication]
Looking for content that’s easy to digest? Check out the press releases below.
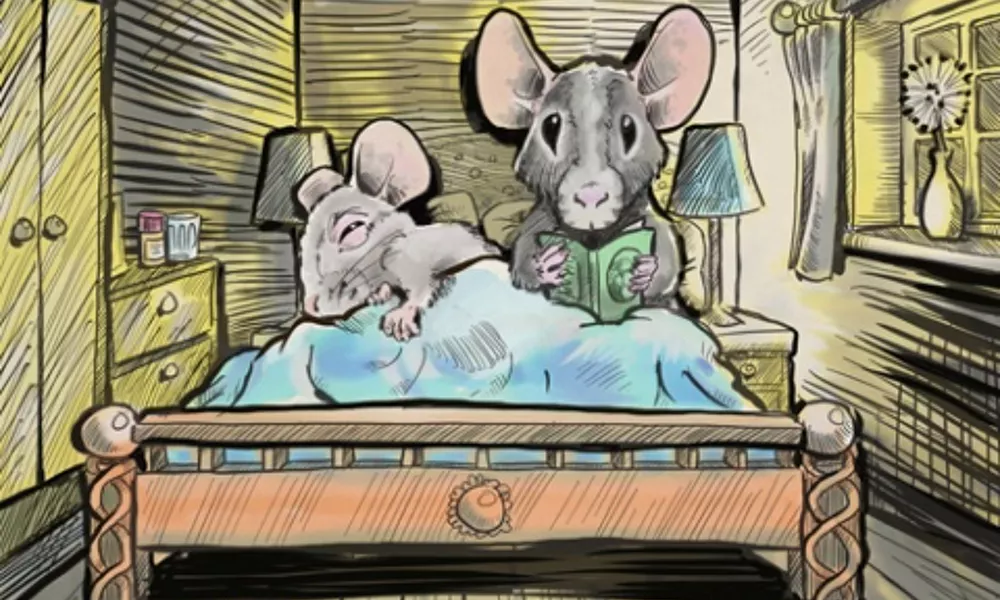
Image: Spencer Phillips/EMBL
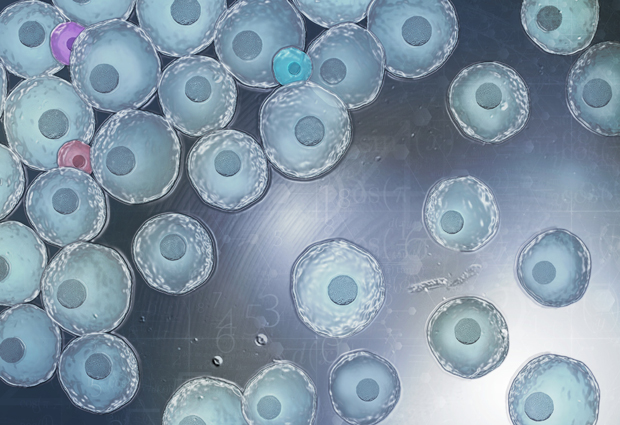
Image: Spencer Phillips/EMBL
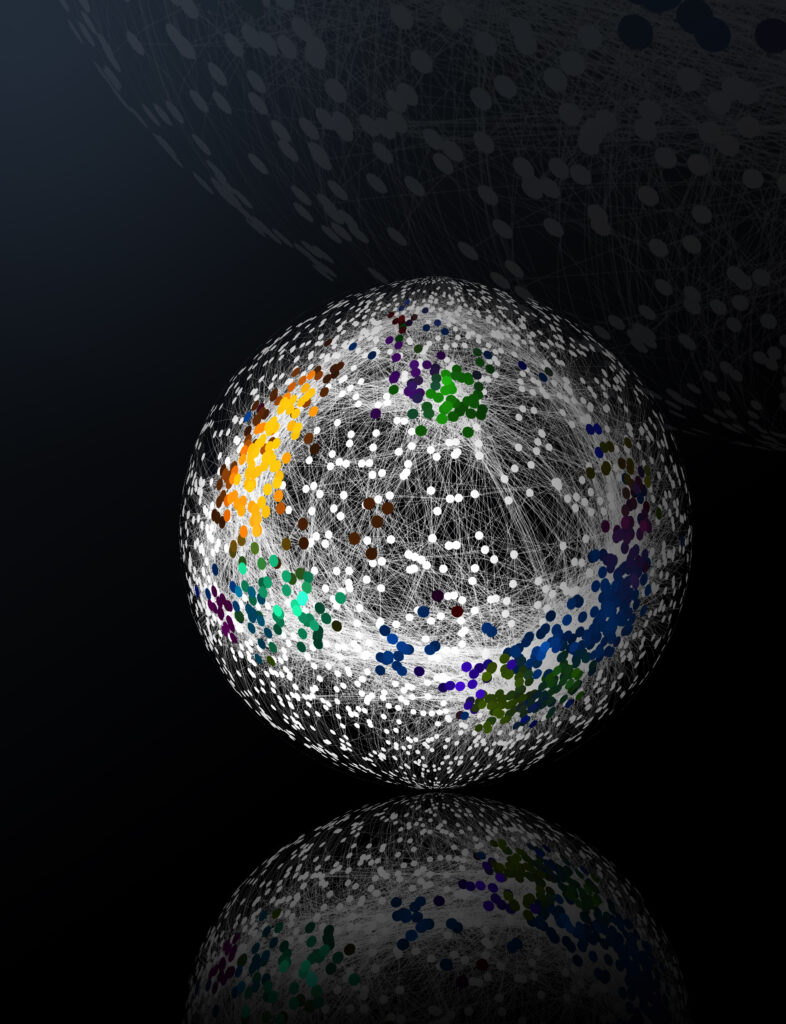
Image: Benedikt Rauscher/DKFZ
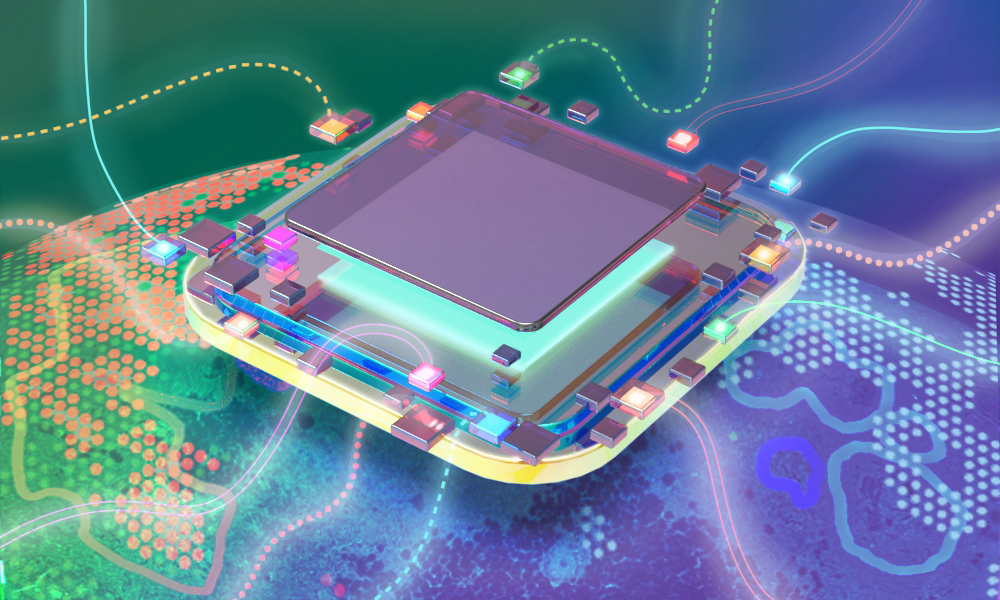
Image: Isabel Romero Calvo/EMBL
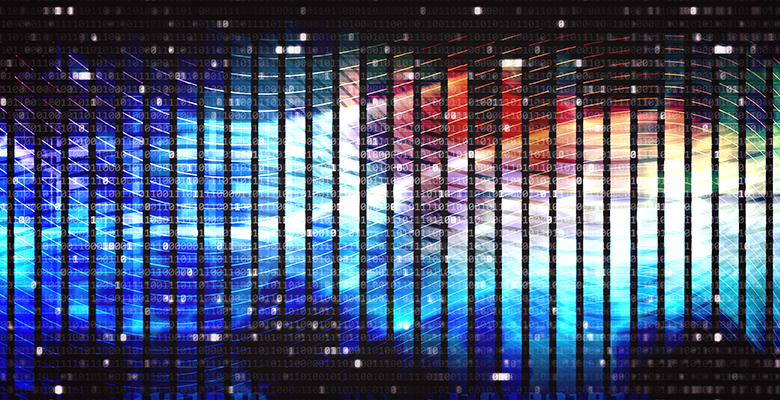
Image: Spencer Phillips/EMBL
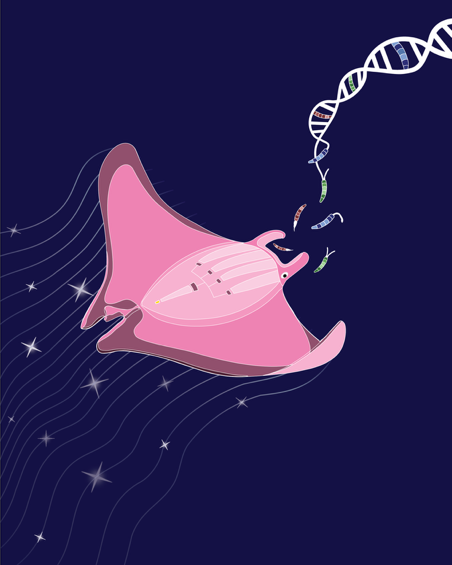
Image: Stegle Lab Members
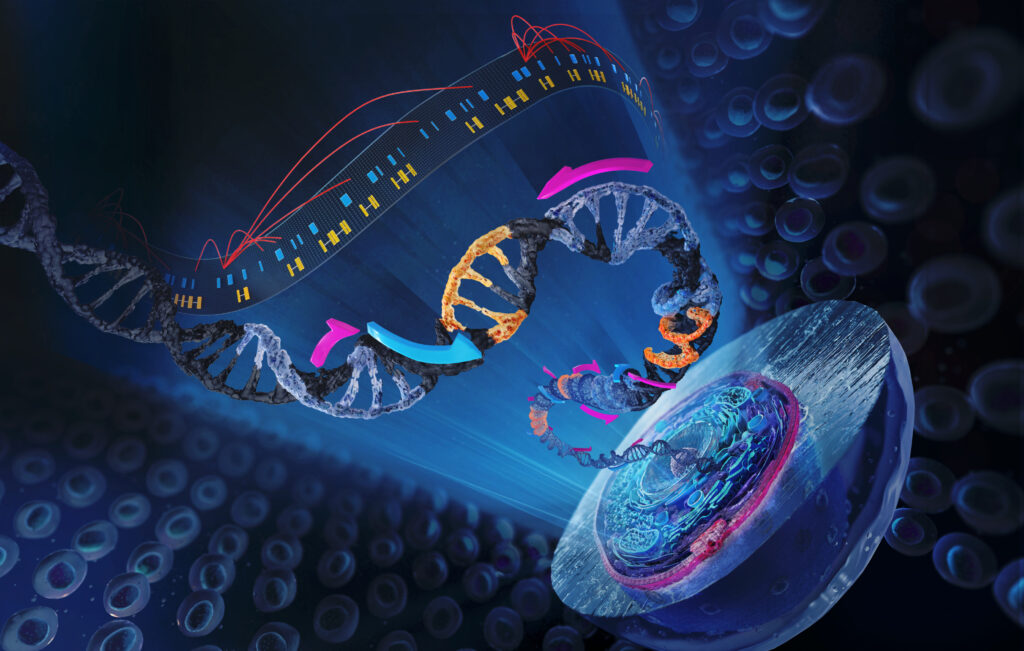
Image: Tobias Wüstefeld/ BlueClay Studios